Amlytic Whitepaper Executive Summary
Collaborative cross-bank Financial Crime Analytics brings a promise of higher detection rates with lower false-positives. However, deploying sophisticated Machine Learning algorithms on larger swaths of data from multiple institutions exposes the limitations of Machine Learning and the complexities of sharing Know-Your-Customer (KYC), Customer Due Diligence (CDD), and other sensitive personal data across multiple institutions.
After decades of a KYC-centric, in-house approach to Anti-Money Laundering (AML), financial institutions incorrectly assume that collaborative cross-bank AML should necessarily involve sharing of sensitive data as well. This assumption clashes with basic principles of data protection and limits the prospects of a truly comprehensive, bulk-scale cross-bank transaction monitoring system.
The emerging field of Privacy Enhancing Technologies (PETs) brings a promise of breaking the data-sharing barrier. However, since GDPR treats even obfuscated personal data as personal data regardless of the level and strength of obfuscation, the concept of truly anonymized, Data Subject-level personal data remains practically unattainable in real-life applications, even with the use of PETs.
Beyond the data sharing challenges, contemporary Machine Learning-based analytics models require accurate labelled data for training and testing, and produce results that cannot always be adequately explained. Given the historical tendency of financial institutions to 'over-report' suspicion, even the most advanced artificial intelligence trained on financial institutions' isolated or pooled data is bound to produce results that reflect the historically poor track record of spotting and reporting complex financial crime patterns.
In this White Paper, we present Amlytic - an alternative, context-centric approach to bulk-scale crossinstitutional Financial Crime analytics at the pre-suspicion level. Amlytic emphasises data minimization on top of anonymization, with privacy considerations built into the platform design from ground-up. Designed to work at bank-scale and viable under the existing legal and data protection frameworks, Amlytic is capable of delivering robust and explainable results by using as little of the underlying shared data as possible, and with no requirement for training data.
The analytical precision of our computational framework was first proven during joint FCA / US-Treasury AML TechSprint in 2019. The same analytical framework was subsequently deployed for the TriBank Initiative PoC in the UK, which demonstrated the merit of pooling real-life pseudonymized transactional data across multiple institutions. In 2022, Amlytic was presented as a comprehensive privacy preserving and analytical platform for the The French Prudential Supervision and Resolution Authority (ACPR) Confidential Data Pooling TechSprint, where it was chosen as the winning solution by a group of participating financial institutions.
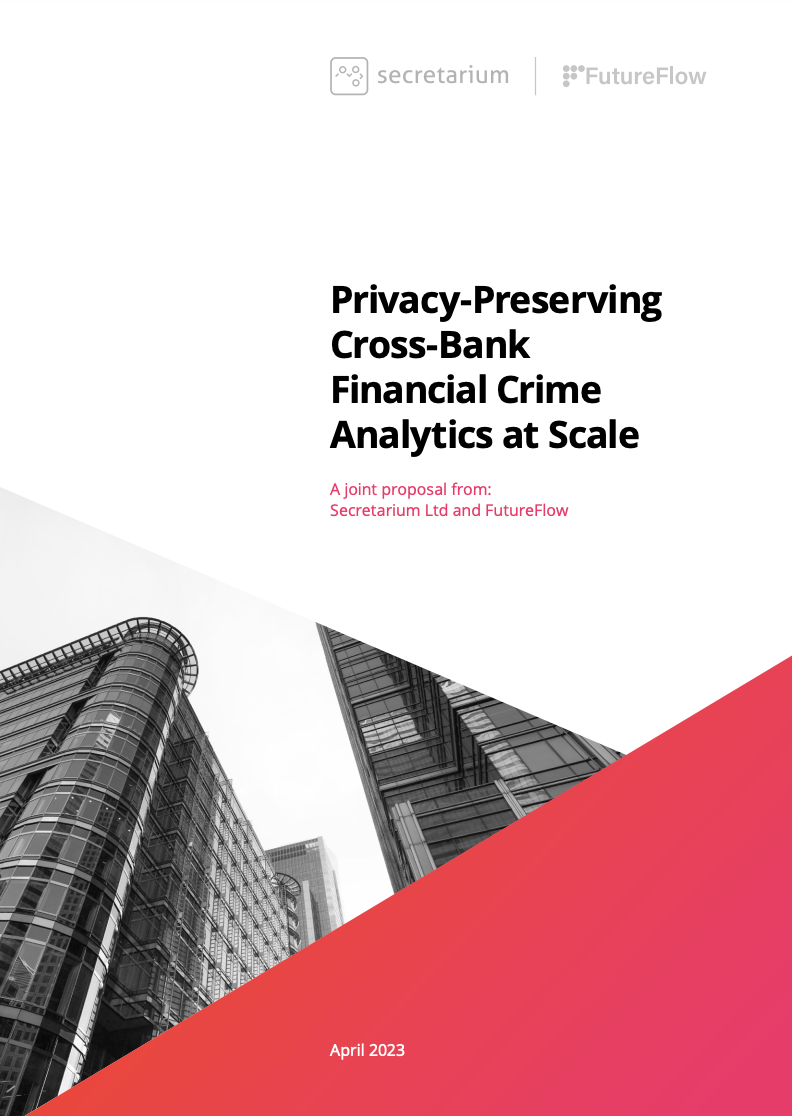
To continue reading the whitepaper, please fill out the form and we will get in touch with you.